Farmers around the world, including in the EU, face many challenges. Their daily work and businesses need to be rethought in order to reduce the negative impact on the environment and achieve a sustainable economy in the long term. For example, with the Green Deal, the EU has issued new restrictions aimed at reducing nitrogen fertilization by 20%. One of the pillars for achieving this goal is digitalization. Digital products have the potential to automate farmers’ work and provide decision support systems that make the farmers’ daily lives easier. However, data quality is often not explicitly considered and its impact on the success of digitalization in agriculture still needs to be investigated. In this Fraunhofer IESE blog post, we give an overview of the current challenges of digitalization in agriculture. We also discuss the benefits of assessing data quality issues and briefly describe how this approach works. We also outline the benefits for farmers of having high-quality data.
For more info on data quality, see:
- Our first blog on the technical solution for data quality in precision farming.
- Also, check our previous post on data quality issues in nutrient cycles here.
Current challenges regarding digitalization in agriculture
There are many digital technology providers in the field of agriculture. Just as the applications vary, so do the individually developed components that provide different and heterogenous data sets and sources. Often, technology vendors use their own Farm Management Information Systems (FMIS), which are incompatible and not interoperable, meaning that the different systems cannot communicate with each other. To take advantage of digitalization, it is also important to provide farmers with digital skills and technologies directly. The key is to overcome existing technological limitations, for example by using Digital Twins.to link systems together to improve performance in various aspects of farming operations.
Addressing challenges in data quality within the DEMETER project
DEMETER is a Horizon 2020 EU project that aims to drive forward digitalization in agriculture in the long term. Hence, one main goal in DEMETER is to provide interoperability between different software and hardware providers and actively involve the relevant end-users, i.e., the farmers.
To this end, the project has created a centralized exchange platform for stakeholders from the agricultural domain, which includes:
- a reference architecture,
- data security and data privacy mechanisms (e.g., secure transfer of sensitive data is ensured and access to unauthorized entities is prevented),
- data analytics services and smart farming services (e.g., decision support systems),
- an ontology combining the terminologies and vocabularies from multiple data standards used by different players in the domain of agriculture. This ontology is called AIM (agricultural information model) and the project plans to standardize this eventually.
Having about 60 partners, the project covers various sub-sectors of agriculture, namely Arable Crops, Precision Farming, Fruits and Vegetables, Livestock, and Supply Chain.
As more and more data is generated, we want to provide farmers with better data quality in the long term. Our contributions to this project therefore include the development of a tool for assessing the quality of agricultural data and analysis of this data to enable precision farming through our involvement in two use cases (see pilot 2.1 and pilot 2.2). In the following, we will demonstrate how the DQA (data quality assessment) tool we developed fits into this context, i.e., how it empowers others to increase their awareness and understanding of their data to drive digitalization in agriculture.
Usage of data quality assessment in agriculture
We developed the Data Quality Assessment tool for structured data to enable data owners or consumers, e.g., farmers, manufacturers, dealers, analytics providers, etc. to automatically check and assess the quality of data in terms of dimensions like completeness, credibility, consistency, and accuracy. Our tool can currently detect 17 kinds of data quality problems and can be easily extended and customized to fit to one’s own context. The tool is an open-source (Apache2) REST-API written in Python, accessible and readable by a machine or through a web-based user interface.
The DQA tool can be integrated into the agriculture workflow as demonstrated in the DEMETER project. First, we assume that the farmer performs an operation using a machine or sensor that collects data. The corresponding raw data is stored in an FMIS or another (cloud) application. This data can be pre-processed or used directly as input for the DQA tool. Our DQA tool calculates several mathematical functions for each of the data quality dimensions listed above and returns the results in the form of ratios or serialized graphics, along with – on demand – a list of problematic entries. This flexibility is made possible by the instantiation of a configuration file, which allows the user to define their own values for the different parameters. The DQA tool can be run locally, i.e., without connecting it to external sources, so that data privacy is guaranteed: Data to be analyzed does not leave the computer or the docker container. It is also possible to access it through its REST-API interface on a remote server. The measurement results are presented in a format that is readable for both humans and machines (JSON).
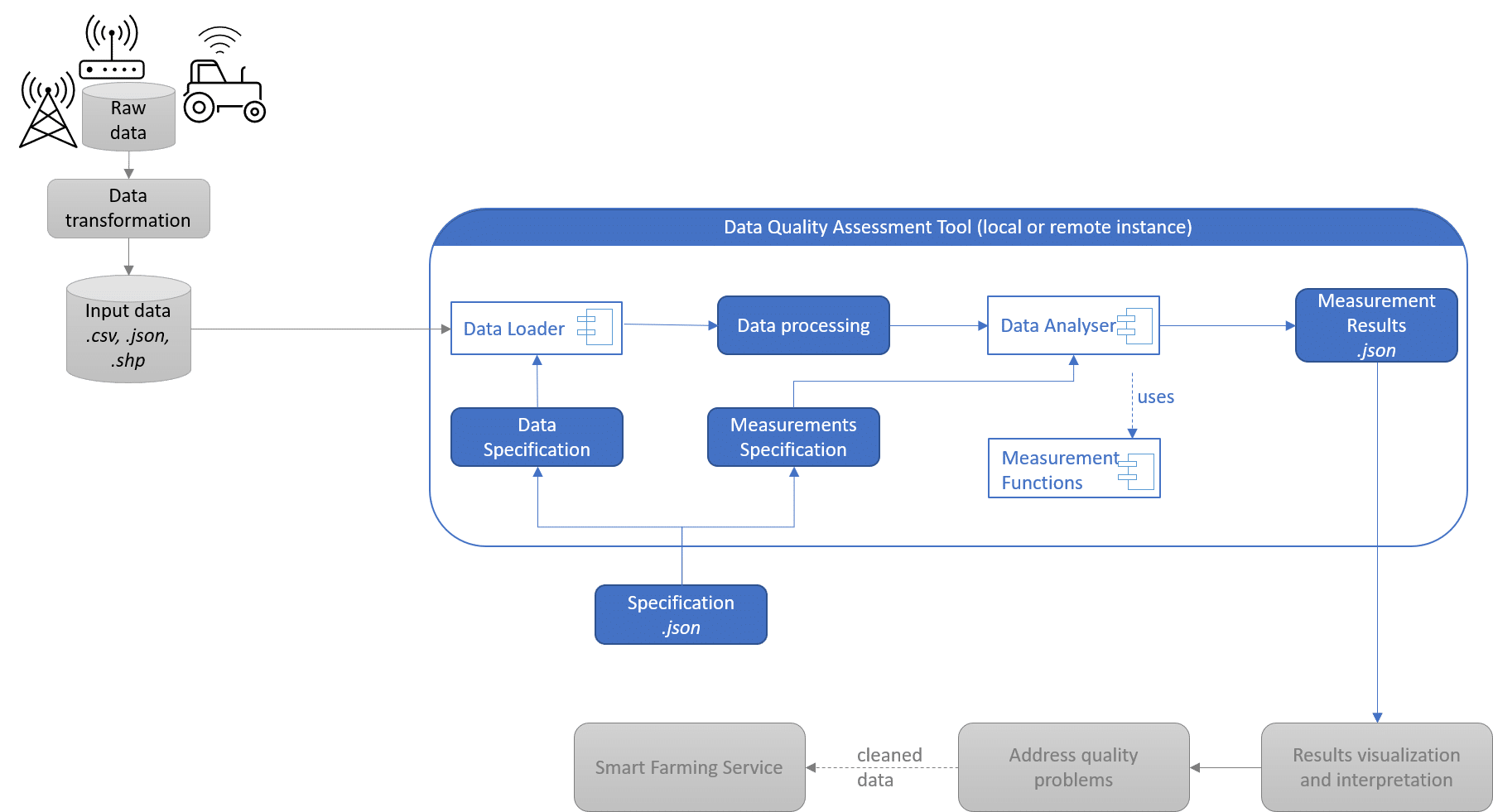
Once the quality issues have been assessed, it is still necessary to understand the source of the problems and to determine whether or not these errors are problematic and how to resolve them. The analysis of the DQA and the “data cleaning” operations must be carried out in an additional step, which again requires both agricultural and technical expertise.
We extended our DQA tool to specifically analyze machinery emissions data for the DEMETER project. This extension provides a graphical evaluation of the data with color ranges, from green (good values) to red (bad values), for a quick analysis of the machine’s condition.
Benefits of data quality assurance
- For farmers: By ensuring the quality of data, farmers can make more reliable decisions about their operations. For example, they can use the data to optimize crop yields, reduce waste, and improve efficiency. Additionally, accurate data can help farmers to better understand the performance of their machinery, leading to more effective maintenance and repairs.
- For developers: The open-source and open-API-compliant nature of our tool allows developers to easily integrate it into their own software systems. The ability to configure the tool through a configuration file and handle multiple file formats (for structured data) provides a high degree of flexibility. This makes it easier for developers to work with the tool and adapt it to their specific needs. The tool is highly configurable to meet specific requirements and can be seamlessly integrated into existing pipelines. Additionally, it produces results that can be easily interpreted by both humans and machines, making it a valuable asset for any data-related task.
- For data owners: The flexibility of being able to run the tool on premises, in a docker container, or remotely provides significant benefits in terms of data privacy and security, as it allows for the data to be kept within the desired environment and not be shared with external parties.
Overall, the use of our data quality assessment tool can lead to increased productivity, reduced costs, and improved outcomes for farmers.
Data quality plays a key role when analyzing or using your data. Are you looking for support in implementing Artificial Intelligence solutions?
Do you want to find out the AI potential for your business in our AI innovation lab?
Contact our expert patricia.kelbert@iese.fraunhofer.de.
References
[1] Schroth, Christof, Patricia Kelbert, and Anna Maria Vollmer. „A data quality assessment tool for agricultural structured data as support for smart farming.“ 43. GIL-Jahrestagung, Resiliente Agri-Food-Systeme (2023).
[2] Abdipourchenarestansofla, Morteza, and Christof Schroth. „The importance of data quality assessment for machinery data in the field of agriculture.“ 79th International Conference on Agricultural Engineering. No. 2395. 2022.